Tail Assignment Optimization for Airlines
Tail Assignment Optimization for Airlines
Tail Assignment Optimization for Airlines
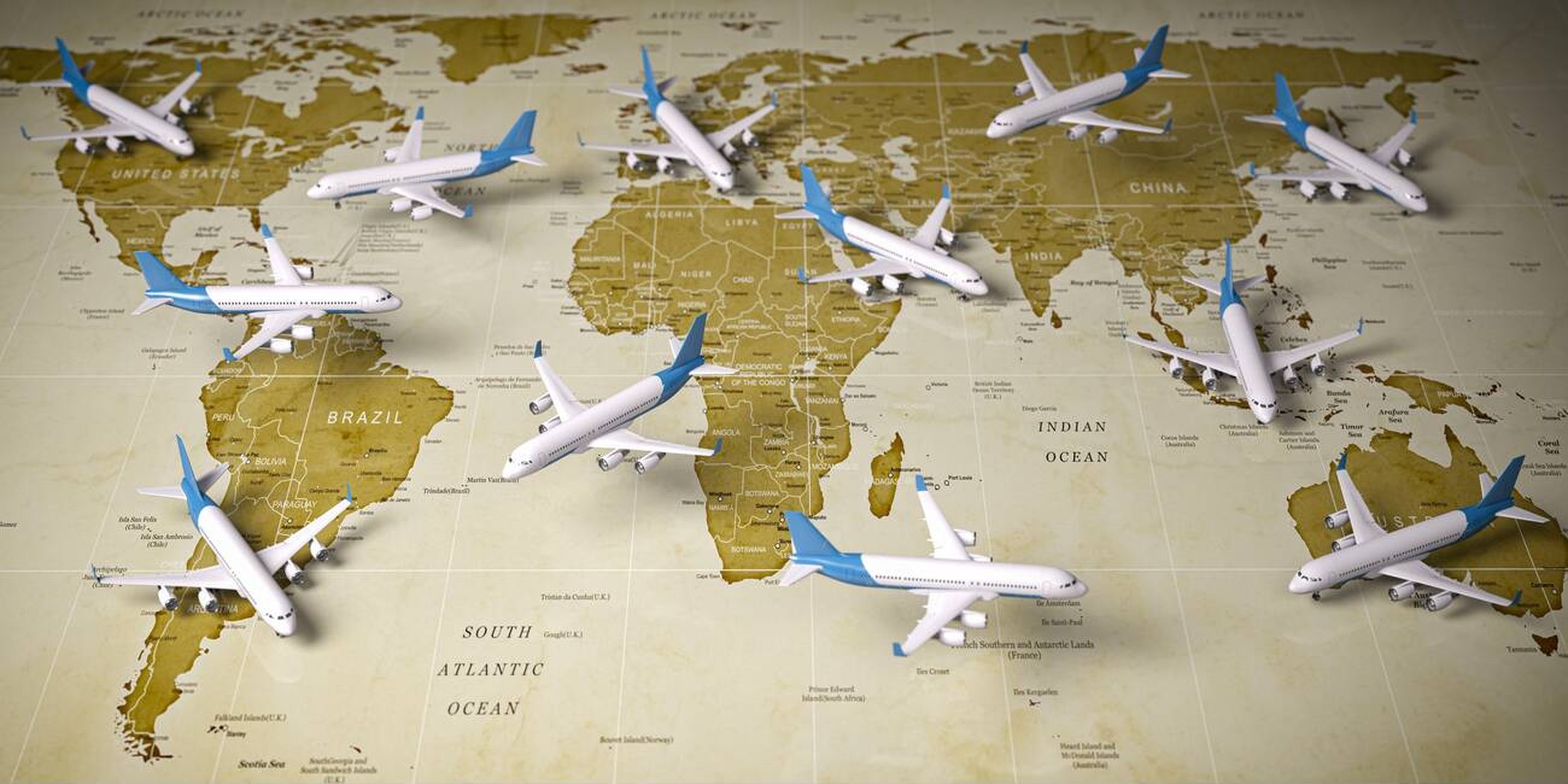
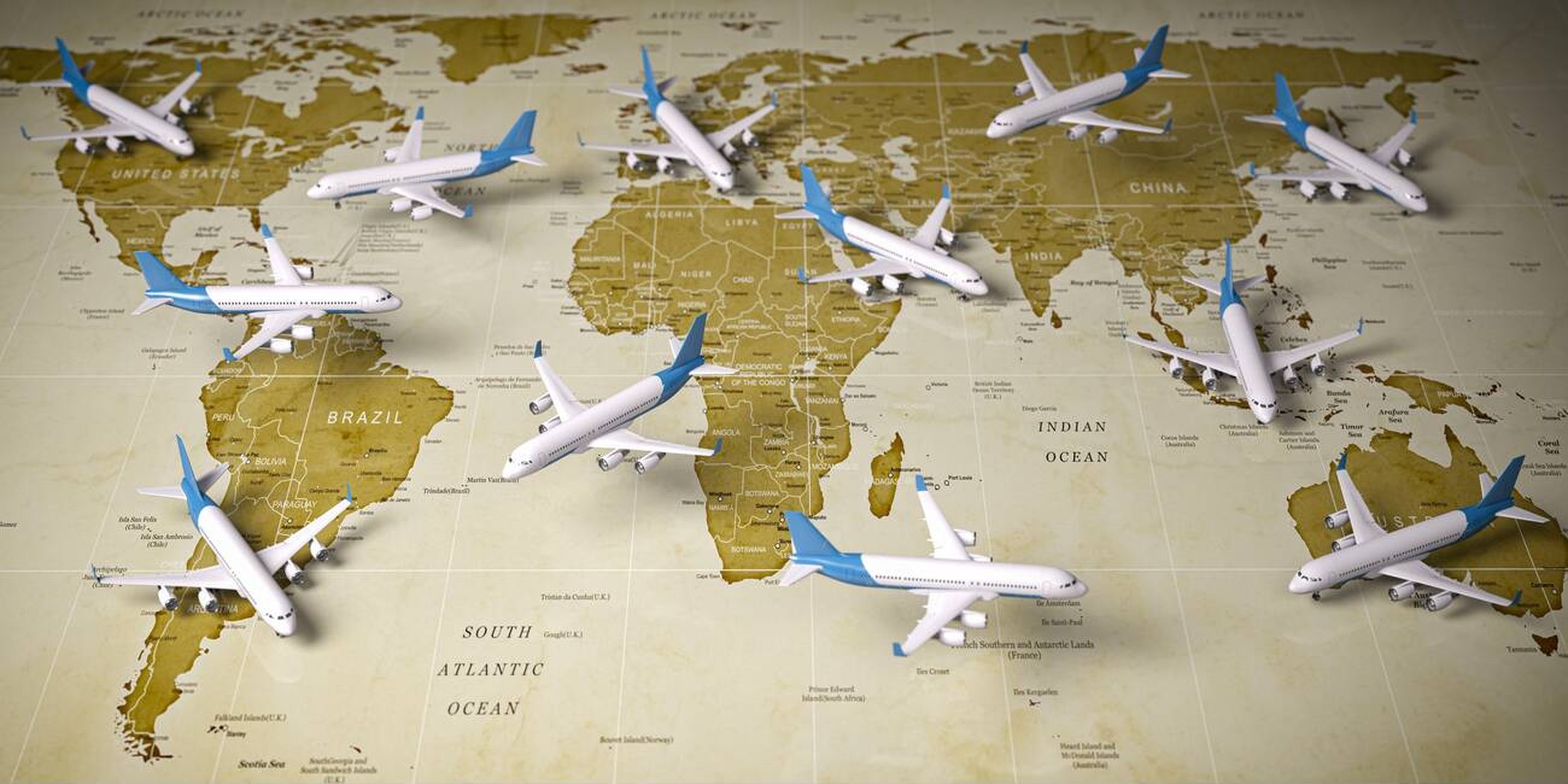
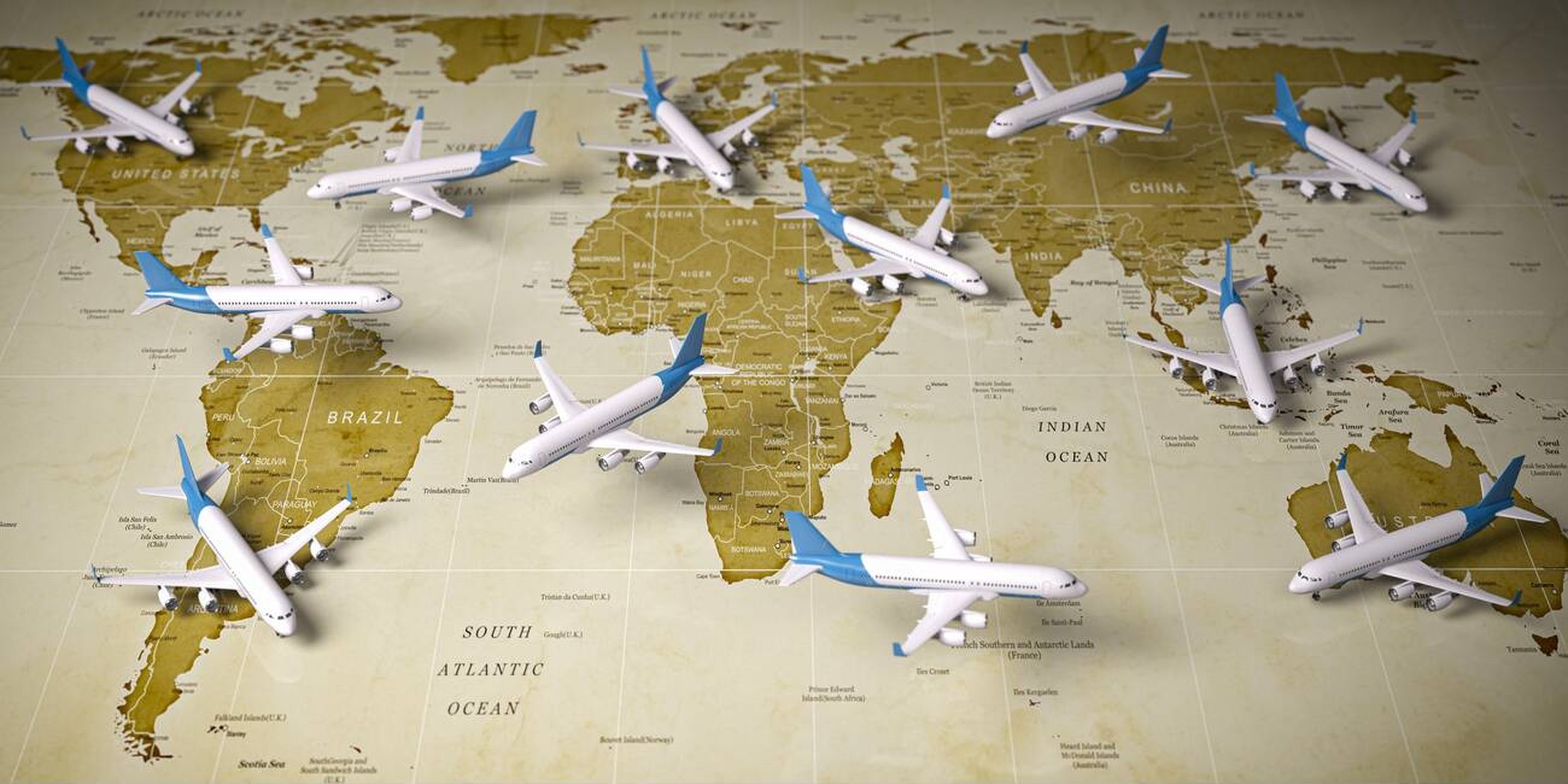
To optimize aircraft tail assignment while maximizing flight coverage and honoring various operational constraints.
To optimize aircraft tail assignment while maximizing flight coverage and honoring various operational constraints.
A middle-east airline encountered significant challenges in managing tail assignments through manual processes. These manual practices led to increased complexity and operational risks as the fleet continued to grow, resulting in inefficiencies and potential scheduling conflicts. To overcome these obstacles, we developed an automated tail assignment optimization system designed to streamline operations and enhance decision-making capabilities. By leveraging advanced optimization techniques, we aimed to improve operational efficiency, reduce risks, and support seamless expansion for the airline.
A middle-east airline encountered significant challenges in managing tail assignments through manual processes. These manual practices led to increased complexity and operational risks as the fleet continued to grow, resulting in inefficiencies and potential scheduling conflicts. To overcome these obstacles, we developed an automated tail assignment optimization system designed to streamline operations and enhance decision-making capabilities. By leveraging advanced optimization techniques, we aimed to improve operational efficiency, reduce risks, and support seamless expansion for the airline.
A middle-east airline encountered significant challenges in managing tail assignments through manual processes. These manual practices led to increased complexity and operational risks as the fleet continued to grow, resulting in inefficiencies and potential scheduling conflicts. To overcome these obstacles, we developed an automated tail assignment optimization system designed to streamline operations and enhance decision-making capabilities. By leveraging advanced optimization techniques, we aimed to improve operational efficiency, reduce risks, and support seamless expansion for the airline.
Results
Results
Streamlined tail assignment process, minimizing manual intervention.
Increased assignment coverage and optimized aircraft utilization.
Reduced unnecessary repositioning, increasing operational efficiency and cost savings.
Accelerated and more informed decision-making.
Streamlined tail assignment process, minimizing manual intervention.
Increased assignment coverage and optimized aircraft utilization.
Reduced unnecessary repositioning, increasing operational efficiency and cost savings.
Accelerated and more informed decision-making.
The implementation process began with a thorough analysis of the airline's existing manual assignment process and its limitations. We then formulated a Mixed Integer Programming (MIP) model that captured all relevant constraints and objectives. This mathematical model was designed to maximize flight coverage while balancing aircraft utilization across the fleet. The model incorporated various constraints ranging from operational requirements to scheduling restrictions and user preferences.
The IBM ILOG CPLEX optimizer was chosen to solve this complex model due to its ability to handle large-scale optimization problems efficiently. The team worked on data integration, ensuring that all necessary inputs - such as flight schedules, aircraft details, airport information, and maintenance schedules - were properly formatted and fed into the model.
A user-friendly interface was developed to allow planners to easily input parameters, run the optimization, and visualize the results. This interface also enabled "what-if" analysis, allowing users to adjust various parameters and quickly see the impact on the overall schedule.
The implementation process began with a thorough analysis of the airline's existing manual assignment process and its limitations. We then formulated a Mixed Integer Programming (MIP) model that captured all relevant constraints and objectives. This mathematical model was designed to maximize flight coverage while balancing aircraft utilization across the fleet. The model incorporated various constraints ranging from operational requirements to scheduling restrictions and user preferences.
The IBM ILOG CPLEX optimizer was chosen to solve this complex model due to its ability to handle large-scale optimization problems efficiently. The team worked on data integration, ensuring that all necessary inputs - such as flight schedules, aircraft details, airport information, and maintenance schedules - were properly formatted and fed into the model.
A user-friendly interface was developed to allow planners to easily input parameters, run the optimization, and visualize the results. This interface also enabled "what-if" analysis, allowing users to adjust various parameters and quickly see the impact on the overall schedule.
The implementation process began with a thorough analysis of the airline's existing manual assignment process and its limitations. We then formulated a Mixed Integer Programming (MIP) model that captured all relevant constraints and objectives. This mathematical model was designed to maximize flight coverage while balancing aircraft utilization across the fleet. The model incorporated various constraints ranging from operational requirements to scheduling restrictions and user preferences.
The IBM ILOG CPLEX optimizer was chosen to solve this complex model due to its ability to handle large-scale optimization problems efficiently. The team worked on data integration, ensuring that all necessary inputs - such as flight schedules, aircraft details, airport information, and maintenance schedules - were properly formatted and fed into the model.
A user-friendly interface was developed to allow planners to easily input parameters, run the optimization, and visualize the results. This interface also enabled "what-if" analysis, allowing users to adjust various parameters and quickly see the impact on the overall schedule.
Solution
Solution
A Mixed Integer Programming (MIP) model was formulated to optimize the entire planning process, considering multiple objectives and constraints. The model was solved using IBM ILOG CPLEX optimizer.
A Mixed Integer Programming (MIP) model was formulated to optimize the entire planning process, considering multiple objectives and constraints. The model was solved using IBM ILOG CPLEX optimizer.
To achieve an optimal aircraft tail assignment solution, the optimization model needed to consider several key constraints.
For instance, maintaining geographic continuity ensured smooth transitions between flight segments, while adhering to ground time requirements guaranteed efficient operations. Onward connectivity was prioritized to facilitate seamless passenger transfers, and balancing aircraft utilization played a vital role in maintaining operational efficiency. The model considered aircraft scheduling preferences and restrictions, while ensuring necessary maintenance activities were performed within designated time frames. Additionally, various operational constraints specific to the airline's need has to be incorporated into the model.
To achieve an optimal aircraft tail assignment solution, the optimization model needed to consider several key constraints.
For instance, maintaining geographic continuity ensured smooth transitions between flight segments, while adhering to ground time requirements guaranteed efficient operations. Onward connectivity was prioritized to facilitate seamless passenger transfers, and balancing aircraft utilization played a vital role in maintaining operational efficiency. The model considered aircraft scheduling preferences and restrictions, while ensuring necessary maintenance activities were performed within designated time frames. Additionally, various operational constraints specific to the airline's need has to be incorporated into the model.
To achieve an optimal aircraft tail assignment solution, the optimization model needed to consider several key constraints.
For instance, maintaining geographic continuity ensured smooth transitions between flight segments, while adhering to ground time requirements guaranteed efficient operations. Onward connectivity was prioritized to facilitate seamless passenger transfers, and balancing aircraft utilization played a vital role in maintaining operational efficiency. The model considered aircraft scheduling preferences and restrictions, while ensuring necessary maintenance activities were performed within designated time frames. Additionally, various operational constraints specific to the airline's need has to be incorporated into the model.
Benefits
Benefits
Cost savings through improved operational efficiency and resource utilization.
Increased employee productivity.
Enhanced competitive advantage.
Improved on-time performance and reduced flight disruptions contributing to a better customer experience.
Better preparedness for fleet expansion.
Cost savings through improved operational efficiency and resource utilization.
Increased employee productivity.
Enhanced competitive advantage.
Improved on-time performance and reduced flight disruptions contributing to a better customer experience.
Better preparedness for fleet expansion.
By implementing tail assignment optimization solution, the airline realized substantial cost savings and enhanced operational efficiency. The model facilitated the creation of schedules for a longer planning horizon, thereby offering the business improved visibility. It further reduced reliance on individual expertise, boosting employee productivity and strengthening competitive advantage. The solution streamlined schedule planning, enhancing preparedness for fleet expansion and better managing disruptions. Adherence to maintenance schedules and regulatory requirements was improved, increasing flexibility and optimizing fleet usage. Ultimately, the model delivered significant time savings, reliability, and on-time performance.
By implementing tail assignment optimization solution, the airline realized substantial cost savings and enhanced operational efficiency. The model facilitated the creation of schedules for a longer planning horizon, thereby offering the business improved visibility. It further reduced reliance on individual expertise, boosting employee productivity and strengthening competitive advantage. The solution streamlined schedule planning, enhancing preparedness for fleet expansion and better managing disruptions. Adherence to maintenance schedules and regulatory requirements was improved, increasing flexibility and optimizing fleet usage. Ultimately, the model delivered significant time savings, reliability, and on-time performance.
By implementing tail assignment optimization solution, the airline realized substantial cost savings and enhanced operational efficiency. The model facilitated the creation of schedules for a longer planning horizon, thereby offering the business improved visibility. It further reduced reliance on individual expertise, boosting employee productivity and strengthening competitive advantage. The solution streamlined schedule planning, enhancing preparedness for fleet expansion and better managing disruptions. Adherence to maintenance schedules and regulatory requirements was improved, increasing flexibility and optimizing fleet usage. Ultimately, the model delivered significant time savings, reliability, and on-time performance.
The implementation of the tail assignment optimization system showcases the transformative potential of advanced analytics in airline operations. By automating manual processes with a constraint-aware solution, airlines can achieve significant improvements in flight coverage, aircraft utilization, and overall operational efficiency.
This adaptable system, powered by mixed integer programming model, can be tailored to other airlines, leading to improved resource utilization, cost savings, and schedule reliability. Embracing such tools is vital for maintaining competitiveness and ensuring a resilient, efficient future for the aviation industry.
As the aviation industry continues to face pressures for efficiency and reliability, such optimization tools will become increasingly crucial for maintaining competitiveness and delivering value to both the airline and its passengers.
The implementation of the tail assignment optimization system showcases the transformative potential of advanced analytics in airline operations. By automating manual processes with a constraint-aware solution, airlines can achieve significant improvements in flight coverage, aircraft utilization, and overall operational efficiency.
This adaptable system, powered by mixed integer programming model, can be tailored to other airlines, leading to improved resource utilization, cost savings, and schedule reliability. Embracing such tools is vital for maintaining competitiveness and ensuring a resilient, efficient future for the aviation industry.
As the aviation industry continues to face pressures for efficiency and reliability, such optimization tools will become increasingly crucial for maintaining competitiveness and delivering value to both the airline and its passengers.
For more information, write to us at info@planoptimus.com